Scientists from Saudi Arabia’s King Fahd University of Petroleum & Minerals have analyzed the benefits of an ensemble-based deep learning framework for PV cell defects classification. Ensemble deep learning combines multiple deep learning models to improve the accuracy of the prediction.
The group tested eight advanced stand-alone models and compared their performance with those of two ensemble techniques known as voting and bagging.
“For the voting technique, we have eight trained models for the voting ensemble, each with unique performance values. The voting aggregation techniques are applied to improve overall performance. In this paper, we used the soft voting technique, which operates on a majority vote using the average performance values of each model,” the team explained. “In bagging ensemble methods, the training dataset is sampled and distributed to the various models, using soft voting aggregation for the performance metric.”
All methods were trained and tested on the ELPV benchmark dataset, which includes 2,624 electroluminescence (EL) images of PV cells. The dataset is categorized into four classes – functional, moderate, mild, and severe defect, and the models were asked to place them in the correct category. In addition, a binary test was also conducted, where the functional and moderate classes were considered non-defective and mild and severe as defective.
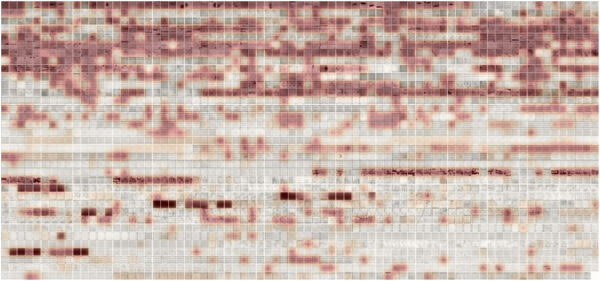
Image: King Fahd University of Petroleum & Minerals, Case Studies in Thermal Engineering, CC BY 4.0
“This study systematically evaluates the performance of popular computer vision architectures—AlexNet, SENet, GoogleNet (Inception V1), Xception, Vision Transformer (ViT), Darknet53, ResNet18, and Squeeze Net—in classifying defects in photovoltaic panels,” the team said. “This study addresses a significant gap in photovoltaic system research by integrating sophisticated defect detection techniques with machine learning ensemble methods, thereby improving the reliability and efficiency of solar energy systems in adverse environmental conditions.”
Per the results, in analyzing four classes of defects, the voting ensemble achieved 68.36% accuracy while bagging had 68.31%. The lowest-performing single model was YOLOv3, with an accuracy of 51.27%, whereas AlexNet had the best single model results, with 67.62%.
According to the binary test results, ResNet18 achieved the highest accuracy of 73.02%, surpassing both voting 72.17% and bagging 72.06%. The lowest single model accuracy under those settings was of ViT, with 39.68%.
The methods were presented in “Solar photovoltaic panel cell defects classification using deep learning ensemble methods,” published in Case Studies in Thermal Engineering.
This content is protected by copyright and may not be reused. If you want to cooperate with us and would like to reuse some of our content, please contact: editors@pv-magazine.com.
1 comment
By submitting this form you agree to pv magazine using your data for the purposes of publishing your comment.
Your personal data will only be disclosed or otherwise transmitted to third parties for the purposes of spam filtering or if this is necessary for technical maintenance of the website. Any other transfer to third parties will not take place unless this is justified on the basis of applicable data protection regulations or if pv magazine is legally obliged to do so.
You may revoke this consent at any time with effect for the future, in which case your personal data will be deleted immediately. Otherwise, your data will be deleted if pv magazine has processed your request or the purpose of data storage is fulfilled.
Further information on data privacy can be found in our Data Protection Policy.