A research group led by France's University of Toulouse has developed a novel detection method for snail trails in solar modules. “In the next stages of our research, we are working on building a formal fault dictionary to enable algorithms that can be applied under multiple conditions and across various technologies,” corresponding author Dr. Edgar Hernando Sepúlveda-Oviedo told pv magazine.
The process uses an ensemble learning framework dubbed ELDIAG to analyze the PV panel's time-frequency characteristics and statistics. It collects data from commercially available optimizers without any need for additional sensors or instrumentation.
“Snail trails are lines of local discoloration that occur on PV panels after long-term usage. The name of this effect originates from the illusion that snails or worms have passed over the PV panel,” explained the team. “They do not significantly reduce panel performance but they are the main cause of serious panel deterioration such as microcracks and delamination and can go so far as to set the panel on fire. Managing to detect almost unnoticeable flaws due to the snail trail fault is really a challenge.”
The new system was tested on a string of 16 PV panels, with power ranging from 205 W to 240 W. Eight panels were used for training, and the other eight were used for validation. In each group, four panels were healthy, and four suffered from snail trials. A Tigo optimizer extracted 25 parameters from each panel.
Two competing dimensionality reduction techniques were used to reduce the number of input variables so the algorithm is more efficient. Namely, they are principal component analysis (PCA) and isometric mapping (Isomap), both of which reduce the number of input parameters from 25 to only three.
After reducing parameters, the data is inputted into a simple ensemble learning framework. The ensemble consists of three deep learning methods and uses a majority vote to output a final detection. At least two algorithms need to detect a panel as faulty for the ensemble to rule it as one.
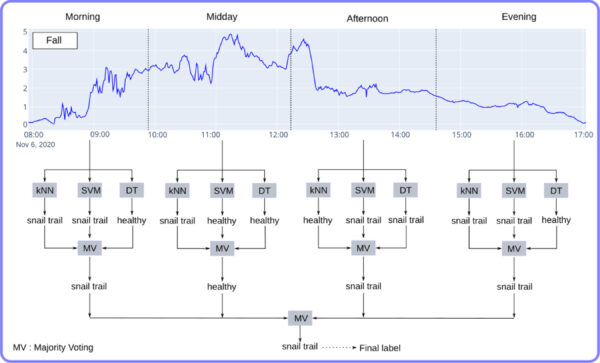
Image: Université de Toulouse, Engineering Applications of Artificial Intelligence, CC BY 4.0
“The component methods of the ensemble learning strategy are K nearest neighbors (KNN), support vector machine (SVM), and decision tree learning (DT),” the group explained. “These three algorithms have been selected because they operate based on three distinct principles, allowing the approach to harness the strengths of each principle. KNN relies on similarity measurement using a distance metric, the SVM method is based on finding an optimal separating hyperplane between two data classes, and, lastly, DT are founded on partitioning a dataset into smaller subsets to enable decision-making based on the information within each subset.”
The signals for both training and validating were taken on four days in 2020, each representing one season. The measurements were taken between 7:00 and 20:00, and results were sliced into morning, midday, afternoon, and evening. While the ELDIAG framework using Isomap feature selection showed accuracy ranging from 70% to 80%, ELDIAG using PCA proved superior, with its accuracy ranging between 79% and 89%.
“Although the accuracy results may not match those achieved by image-based approaches, it is important to highlight the following aspects,” the group said. “Firstly, our approach robustly identifies snail trail faults under varying irradiation conditions using only one current signal per PV panel. This significantly reduces the required number of samples while maintaining the robustness of fault detection. Additionally, our approach does not require any additional installation to function. In economic terms, it is superior, as it offers an industrially scalable solution. Furthermore, by operating using electrical signals, it can detect a large number of faults, surpassing the limitations of image-based approaches.”
The novel system was presented in “An ensemble learning framework for snail trail fault detection and diagnosis in photovoltaic modules,” published in Engineering Applications of Artificial Intelligence. Scientists from France's Université de Toulouse PV equipment supplier Feedgy have conducted the study.
This content is protected by copyright and may not be reused. If you want to cooperate with us and would like to reuse some of our content, please contact: editors@pv-magazine.com.
By submitting this form you agree to pv magazine using your data for the purposes of publishing your comment.
Your personal data will only be disclosed or otherwise transmitted to third parties for the purposes of spam filtering or if this is necessary for technical maintenance of the website. Any other transfer to third parties will not take place unless this is justified on the basis of applicable data protection regulations or if pv magazine is legally obliged to do so.
You may revoke this consent at any time with effect for the future, in which case your personal data will be deleted immediately. Otherwise, your data will be deleted if pv magazine has processed your request or the purpose of data storage is fulfilled.
Further information on data privacy can be found in our Data Protection Policy.